共 16 条
Quantitative Analysis of Coal Properties Using Laser-Induced Breakdown Spectroscopy and Semi-Supervised Learning
被引:0
|作者:
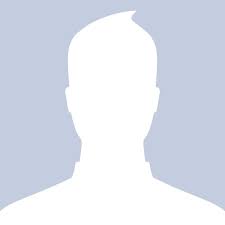
Wang An
论文数: 0 引用数: 0
h-index: 0
机构:
Guodian Changzhou Power Generating Co Ltd, Changzhou 213001, Jiangsu, Peoples R China Guodian Changzhou Power Generating Co Ltd, Changzhou 213001, Jiangsu, Peoples R China
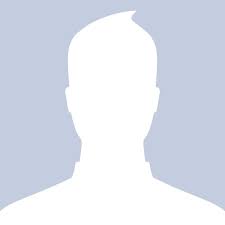
Cui Jia-cheng
论文数: 0 引用数: 0
h-index: 0
机构:
Tsinghua Univ, Int Joint Lab Low Carbon Clean Energy Innovat, Dept Energy & Power Engn, State Key Lab Power Syst, Beijing 100084, Peoples R China Guodian Changzhou Power Generating Co Ltd, Changzhou 213001, Jiangsu, Peoples R China
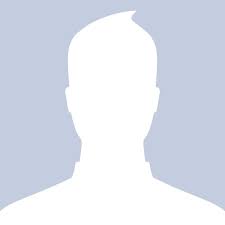
Song Wei-ran
论文数: 0 引用数: 0
h-index: 0
机构:
Tsinghua Univ, Int Joint Lab Low Carbon Clean Energy Innovat, Dept Energy & Power Engn, State Key Lab Power Syst, Beijing 100084, Peoples R China Guodian Changzhou Power Generating Co Ltd, Changzhou 213001, Jiangsu, Peoples R China
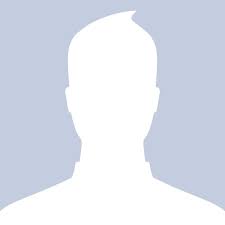
Hou Zong-yu
论文数: 0 引用数: 0
h-index: 0
机构:
Tsinghua Univ, Int Joint Lab Low Carbon Clean Energy Innovat, Dept Energy & Power Engn, State Key Lab Power Syst, Beijing 100084, Peoples R China
Tsinghua Univ, Shanxi Res Inst Clean Energy, Taiyuan 030032, Peoples R China Guodian Changzhou Power Generating Co Ltd, Changzhou 213001, Jiangsu, Peoples R China
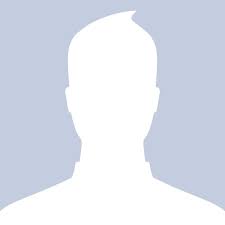
Chen Xiang
论文数: 0 引用数: 0
h-index: 0
机构:
Nanjing Guodian Environm Protect Technol Co Ltd, Nanjing 210061, Peoples R China Guodian Changzhou Power Generating Co Ltd, Changzhou 213001, Jiangsu, Peoples R China
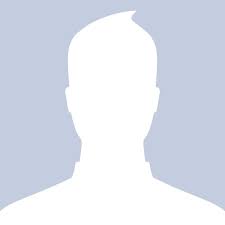
Chen Fei
论文数: 0 引用数: 0
h-index: 0
机构:
Nanjing Guodian Environm Protect Technol Co Ltd, Nanjing 210061, Peoples R China Guodian Changzhou Power Generating Co Ltd, Changzhou 213001, Jiangsu, Peoples R China
机构:
[1] Guodian Changzhou Power Generating Co Ltd, Changzhou 213001, Jiangsu, Peoples R China
[2] Tsinghua Univ, Int Joint Lab Low Carbon Clean Energy Innovat, Dept Energy & Power Engn, State Key Lab Power Syst, Beijing 100084, Peoples R China
[3] Tsinghua Univ, Shanxi Res Inst Clean Energy, Taiyuan 030032, Peoples R China
[4] Nanjing Guodian Environm Protect Technol Co Ltd, Nanjing 210061, Peoples R China
关键词:
Laser-induced breakdown spectroscopy;
Semi-supervised learning;
Coal;
Quantitative analysis;
CARBON;
PLASMA;
D O I:
10.3964/j.issn.1000-0593(2024)07-1940-06
中图分类号:
O433 [光谱学];
学科分类号:
0703 ;
070302 ;
摘要:
Laser-induced breakdown spectroscopy (LIBS) is an emerging atomic spectroscopy technique that has the advantages of low sample pre-treatment and rapid, in situ, and simultaneous multi-element measurements. LIBS demonstrates good prospects in the field of coal analysis. In recent years, chemometric and machine learning models have been widely used to improve the quantitative accuracy of LIBS in coal analysis. Generally, these models rely on a certain number of training samples to ensure the reliability of the prediction results. However, obtaining the certified content (label information) of coal samples used for model training requires traditional chemical analysis, which is complex and time-consuming. This may lead to insufficient training samples and poor model performance. To tackle the small sample problem in LIBS-based coal analysis, this work proposes a semi-supervised learning method based on the ensemble of multiple models. 5 baseline models are first established based on the initial training set, including multiple linear regression (MLR), partial least squares regression (PLSR), locally weighted partial least squares regression (LW-PLSR), support vector regression (SVR), and kernel extreme learning machine (K-ELM). The unlabelled data are processed using the 5 models, and 5 prediction values are obtained. For each unlabelled sample, the standard deviation of the 5 prediction values is calculated, and the unlabelled sample corresponding to the smallest standard deviation is added to the training set. Its pseudo label is the average of the 5 prediction values. As the training set is iteratively expanded, its corresponding training model is updated. The final training model is optimized and used to analyse the test samples. The proposed method is tested on a coal dataset containing 20 training samples, 39 test samples and 280 unlabelled samples. The results show that the proposed method improves the coefficient of determination (R-2) for content prediction of fixed carbon, ash,and volatile by 0.033, 0.102 and 0.118, respectively. Therefore, if the number of training samples is insufficient, semi-supervised learning can effectively improve the accuracy and reliability of LIBS quantification.
引用
收藏
页码:1940 / 1945
页数:6
相关论文
共 16 条
- [1] A comparative model combining carbon atomic and molecular emissions based on partial least squares and support vector regression correction for carbon analysis in coal using LIBS[J]. JOURNAL OF ANALYTICAL ATOMIC SPECTROMETRY, 2019, 34 (03) : 480 - 488Dong, Meirong论文数: 0 引用数: 0 h-index: 0机构: South China Univ Technol, Sch Elect Power, Guangzhou 510640, Guangdong, Peoples R China Guangdong Prov Engn Res Ctr High Efficient & Low, Guangzhou 510640, Guangdong, Peoples R China South China Univ Technol, Sch Elect Power, Guangzhou 510640, Guangdong, Peoples R ChinaWei, Liping论文数: 0 引用数: 0 h-index: 0机构: South China Univ Technol, Sch Elect Power, Guangzhou 510640, Guangdong, Peoples R China Guangdong Prov Engn Res Ctr High Efficient & Low, Guangzhou 510640, Guangdong, Peoples R China South China Univ Technol, Sch Elect Power, Guangzhou 510640, Guangdong, Peoples R ChinaLu, Jidong论文数: 0 引用数: 0 h-index: 0机构: South China Univ Technol, Sch Elect Power, Guangzhou 510640, Guangdong, Peoples R China Guangdong Prov Engn Res Ctr High Efficient & Low, Guangzhou 510640, Guangdong, Peoples R China South China Univ Technol, Sch Elect Power, Guangzhou 510640, Guangdong, Peoples R ChinaLi, Wenbin论文数: 0 引用数: 0 h-index: 0机构: South China Univ Technol, Sch Elect Power, Guangzhou 510640, Guangdong, Peoples R China Guangdong Prov Engn Res Ctr High Efficient & Low, Guangzhou 510640, Guangdong, Peoples R China South China Univ Technol, Sch Elect Power, Guangzhou 510640, Guangdong, Peoples R ChinaLu, Shengzi论文数: 0 引用数: 0 h-index: 0机构: South China Univ Technol, Sch Elect Power, Guangzhou 510640, Guangdong, Peoples R China Guangdong Prov Engn Res Ctr High Efficient & Low, Guangzhou 510640, Guangdong, Peoples R China South China Univ Technol, Sch Elect Power, Guangzhou 510640, Guangdong, Peoples R ChinaLi, Shishi论文数: 0 引用数: 0 h-index: 0机构: South China Univ Technol, Sch Elect Power, Guangzhou 510640, Guangdong, Peoples R China Guangdong Prov Engn Res Ctr High Efficient & Low, Guangzhou 510640, Guangdong, Peoples R China South China Univ Technol, Sch Elect Power, Guangzhou 510640, Guangdong, Peoples R ChinaLiu, Chunyi论文数: 0 引用数: 0 h-index: 0机构: Appl Spectra Inc, 46665 Fremont Blvd, Fremont, CA 94538 USA South China Univ Technol, Sch Elect Power, Guangzhou 510640, Guangdong, Peoples R ChinaYoo, Jong H.论文数: 0 引用数: 0 h-index: 0机构: Appl Spectra Inc, 46665 Fremont Blvd, Fremont, CA 94538 USA South China Univ Technol, Sch Elect Power, Guangzhou 510640, Guangdong, Peoples R China
- [2] Plasma modulation using beam shaping to improve signal quality for laser induced breakdown spectroscopy[J]. JOURNAL OF ANALYTICAL ATOMIC SPECTROMETRY, 2020, 35 (08) : 1671 - 1677Hou, Zongyu论文数: 0 引用数: 0 h-index: 0机构: Tsinghua Univ, Int Joint Lab Low Carbon Clean Energy Innovat, State Key Lab Power Syst, Dept Energy & Power Engn, Beijing 100084, Peoples R China Tsinghua Univ, Int Joint Lab Low Carbon Clean Energy Innovat, State Key Lab Power Syst, Dept Energy & Power Engn, Beijing 100084, Peoples R ChinaAfgan, Muhammad Sher论文数: 0 引用数: 0 h-index: 0机构: Tsinghua Univ, Int Joint Lab Low Carbon Clean Energy Innovat, State Key Lab Power Syst, Dept Energy & Power Engn, Beijing 100084, Peoples R China Tsinghua Univ, Int Joint Lab Low Carbon Clean Energy Innovat, State Key Lab Power Syst, Dept Energy & Power Engn, Beijing 100084, Peoples R ChinaSheta, Sahar论文数: 0 引用数: 0 h-index: 0机构: Tsinghua Univ, Int Joint Lab Low Carbon Clean Energy Innovat, State Key Lab Power Syst, Dept Energy & Power Engn, Beijing 100084, Peoples R China Tsinghua Univ, Int Joint Lab Low Carbon Clean Energy Innovat, State Key Lab Power Syst, Dept Energy & Power Engn, Beijing 100084, Peoples R ChinaLiu, Jiacen论文数: 0 引用数: 0 h-index: 0机构: Tsinghua Univ, Int Joint Lab Low Carbon Clean Energy Innovat, State Key Lab Power Syst, Dept Energy & Power Engn, Beijing 100084, Peoples R China Tsinghua Univ, Int Joint Lab Low Carbon Clean Energy Innovat, State Key Lab Power Syst, Dept Energy & Power Engn, Beijing 100084, Peoples R ChinaWang, Zhe论文数: 0 引用数: 0 h-index: 0机构: Tsinghua Univ, Int Joint Lab Low Carbon Clean Energy Innovat, State Key Lab Power Syst, Dept Energy & Power Engn, Beijing 100084, Peoples R China Tsinghua Univ, Int Joint Lab Low Carbon Clean Energy Innovat, State Key Lab Power Syst, Dept Energy & Power Engn, Beijing 100084, Peoples R China
- [3] A review of artificial neural network based chemometrics applied in laser-induced breakdown spectroscopy analysis[J]. SPECTROCHIMICA ACTA PART B-ATOMIC SPECTROSCOPY, 2021, 180Li, Lu-Ning论文数: 0 引用数: 0 h-index: 0机构: Chinese Acad Sci, Shanghai Inst Tech Phys, Key Lab Space Act Optoelect Technol, Shanghai 200083, Peoples R China Chinese Acad Sci, Shanghai Inst Tech Phys, Key Lab Space Act Optoelect Technol, Shanghai 200083, Peoples R ChinaLiu, Xiang-Feng论文数: 0 引用数: 0 h-index: 0机构: Chinese Acad Sci, Shanghai Inst Tech Phys, Key Lab Space Act Optoelect Technol, Shanghai 200083, Peoples R China Chinese Acad Sci, Shanghai Inst Tech Phys, Key Lab Space Act Optoelect Technol, Shanghai 200083, Peoples R ChinaYang, Fan论文数: 0 引用数: 0 h-index: 0机构: Chinese Acad Sci, Shanghai Inst Tech Phys, Key Lab Space Act Optoelect Technol, Shanghai 200083, Peoples R China Chinese Acad Sci, Shanghai Inst Tech Phys, Key Lab Space Act Optoelect Technol, Shanghai 200083, Peoples R ChinaXu, Wei-Ming论文数: 0 引用数: 0 h-index: 0机构: Chinese Acad Sci, Shanghai Inst Tech Phys, Key Lab Space Act Optoelect Technol, Shanghai 200083, Peoples R China Chinese Acad Sci, Shanghai Inst Tech Phys, Key Lab Space Act Optoelect Technol, Shanghai 200083, Peoples R ChinaWang, Jian-Yu论文数: 0 引用数: 0 h-index: 0机构: Chinese Acad Sci, Shanghai Inst Tech Phys, Key Lab Space Act Optoelect Technol, Shanghai 200083, Peoples R China State Key Lab Infrared Phys, Shanghai 200083, Peoples R China Chinese Acad Sci, Shanghai Inst Tech Phys, Key Lab Space Act Optoelect Technol, Shanghai 200083, Peoples R ChinaShu, Rong论文数: 0 引用数: 0 h-index: 0机构: Chinese Acad Sci, Shanghai Inst Tech Phys, Key Lab Space Act Optoelect Technol, Shanghai 200083, Peoples R China Univ Chinese Acad Sci, Hangzhou Inst Adv Study, Hangzhou 310024, Peoples R China Chinese Acad Sci, Shanghai Inst Tech Phys, Key Lab Space Act Optoelect Technol, Shanghai 200083, Peoples R China
- [4] Improved measurement of the calorific value of pulverized coal particle flow by laser-induced breakdown spectroscopy (LIBS)[J]. ANALYTICAL METHODS, 2019, 11 (35) : 4471 - 4480Li, Wenbing论文数: 0 引用数: 0 h-index: 0机构: South China Univ Technol, Sch Elect Power, Guangzhou 510640, Guangdong, Peoples R China Guangdong Prov Engn Res Ctr High Efficient & Low, Guangzhou 510640, Guangdong, Peoples R China South China Univ Technol, Sch Elect Power, Guangzhou 510640, Guangdong, Peoples R ChinaDong, Meirong论文数: 0 引用数: 0 h-index: 0机构: South China Univ Technol, Sch Elect Power, Guangzhou 510640, Guangdong, Peoples R China Guangdong Prov Engn Res Ctr High Efficient & Low, Guangzhou 510640, Guangdong, Peoples R China South China Univ Technol, Sch Elect Power, Guangzhou 510640, Guangdong, Peoples R ChinaLu, Shengzi论文数: 0 引用数: 0 h-index: 0机构: South China Univ Technol, Sch Elect Power, Guangzhou 510640, Guangdong, Peoples R China Guangdong Prov Engn Res Ctr High Efficient & Low, Guangzhou 510640, Guangdong, Peoples R China South China Univ Technol, Sch Elect Power, Guangzhou 510640, Guangdong, Peoples R ChinaLi, Shishi论文数: 0 引用数: 0 h-index: 0机构: South China Univ Technol, Sch Elect Power, Guangzhou 510640, Guangdong, Peoples R China Guangdong Prov Engn Res Ctr High Efficient & Low, Guangzhou 510640, Guangdong, Peoples R China South China Univ Technol, Sch Elect Power, Guangzhou 510640, Guangdong, Peoples R ChinaWei, Liping论文数: 0 引用数: 0 h-index: 0机构: South China Univ Technol, Sch Elect Power, Guangzhou 510640, Guangdong, Peoples R China Guangdong Prov Engn Res Ctr High Efficient & Low, Guangzhou 510640, Guangdong, Peoples R China South China Univ Technol, Sch Elect Power, Guangzhou 510640, Guangdong, Peoples R ChinaHuang, Jianwei论文数: 0 引用数: 0 h-index: 0机构: South China Univ Technol, Sch Elect Power, Guangzhou 510640, Guangdong, Peoples R China Guangdong Prov Engn Res Ctr High Efficient & Low, Guangzhou 510640, Guangdong, Peoples R China South China Univ Technol, Sch Elect Power, Guangzhou 510640, Guangdong, Peoples R ChinaLu, Jidong论文数: 0 引用数: 0 h-index: 0机构: South China Univ Technol, Sch Elect Power, Guangzhou 510640, Guangdong, Peoples R China Guangdong Univ Petrochem Technol, Coll Mech & Elect Engn, Maoming 525000, Guangdong, Peoples R China Guangdong Prov Engn Res Ctr High Efficient & Low, Guangzhou 510640, Guangdong, Peoples R China South China Univ Technol, Sch Elect Power, Guangzhou 510640, Guangdong, Peoples R China
- [5] Semi-supervised LIBS quantitative analysis method based on co-training regression model with selection of effective unlabeled samples[J]. PLASMA SCIENCE & TECHNOLOGY, 2019, 21 (03)Li, Xiaomeng论文数: 0 引用数: 0 h-index: 0机构: Univ Sci & Technol Beijing, Sch Mech Engn, Beijing 100083, Peoples R China Univ Sci & Technol Beijing, Sch Mech Engn, Beijing 100083, Peoples R ChinaLu, Huili论文数: 0 引用数: 0 h-index: 0机构: Univ Sci & Technol Beijing, Basic Expt Ctr Nat Sci, Beijing 100083, Peoples R China Univ Sci & Technol Beijing, Sch Mech Engn, Beijing 100083, Peoples R ChinaYang, Jianhong论文数: 0 引用数: 0 h-index: 0机构: Univ Sci & Technol Beijing, Sch Mech Engn, Beijing 100083, Peoples R China Univ Sci & Technol Beijing, Sch Mech Engn, Beijing 100083, Peoples R ChinaChang, Fu论文数: 0 引用数: 0 h-index: 0机构: Univ Sci & Technol Beijing, Sch Mech Engn, Beijing 100083, Peoples R China Univ Sci & Technol Beijing, Sch Mech Engn, Beijing 100083, Peoples R China
- [6] A large depth of field LIBS measuring system for elemental analysis of moving samples of raw coal[J]. SPECTROCHIMICA ACTA PART B-ATOMIC SPECTROSCOPY, 2016, 116 : 46 - 50Redoglio, D.论文数: 0 引用数: 0 h-index: 0机构: Univ Milan, Dept Phys, Via Celoria 16, I-20134 Milan, Italy Univ Milan, Dept Phys, Via Celoria 16, I-20134 Milan, ItalyGolinelli, E.论文数: 0 引用数: 0 h-index: 0机构: RSE, T&D Technol Dept, Via Rubattino 54, I-20134 Milan, Italy Univ Milan, Dept Phys, Via Celoria 16, I-20134 Milan, ItalyMusazzi, S.论文数: 0 引用数: 0 h-index: 0机构: RSE, T&D Technol Dept, Via Rubattino 54, I-20134 Milan, Italy Univ Milan, Dept Phys, Via Celoria 16, I-20134 Milan, ItalyPerini, U.论文数: 0 引用数: 0 h-index: 0机构: RSE, T&D Technol Dept, Via Rubattino 54, I-20134 Milan, Italy Univ Milan, Dept Phys, Via Celoria 16, I-20134 Milan, ItalyBarberis, F.论文数: 0 引用数: 0 h-index: 0机构: RSE, T&D Technol Dept, Via Rubattino 54, I-20134 Milan, Italy Univ Milan, Dept Phys, Via Celoria 16, I-20134 Milan, Italy
- [7] Coal analysis by laser-induced breakdown spectroscopy: a tutorial review[J]. JOURNAL OF ANALYTICAL ATOMIC SPECTROMETRY, 2019, 34 (06) : 1047 - 1082Sheta, Sahar论文数: 0 引用数: 0 h-index: 0机构: Tsinghua Univ, Dept Energy & Power Engn, State Key Lab Power Syst, Beijing 100084, Peoples R China Cairo Univ, Natl Inst Laser Enhanced Sci, Cairo 12613, Egypt Tsinghua Univ, Dept Energy & Power Engn, State Key Lab Power Syst, Beijing 100084, Peoples R ChinaAfgan, Muhammad Sher论文数: 0 引用数: 0 h-index: 0机构: Tsinghua Univ, Dept Energy & Power Engn, State Key Lab Power Syst, Beijing 100084, Peoples R China Tsinghua Univ, Dept Energy & Power Engn, State Key Lab Power Syst, Beijing 100084, Peoples R ChinaHou, Zongyu论文数: 0 引用数: 0 h-index: 0机构: Tsinghua Univ, Dept Energy & Power Engn, State Key Lab Power Syst, Beijing 100084, Peoples R China Tsinghua Univ, Dept Energy & Power Engn, State Key Lab Power Syst, Beijing 100084, Peoples R ChinaYao, Shun-Chun论文数: 0 引用数: 0 h-index: 0机构: South China Univ Technol, Sch Elect Power, Guangzhou 510640, Guangdong, Peoples R China Tsinghua Univ, Dept Energy & Power Engn, State Key Lab Power Syst, Beijing 100084, Peoples R ChinaZhang, Lei论文数: 0 引用数: 0 h-index: 0机构: Shanxi Univ, Inst Laser Spect, State Key Lab Quantum Opt & Quantum Opt Devices, Taiyuan 030006, Shanxi, Peoples R China Tsinghua Univ, Dept Energy & Power Engn, State Key Lab Power Syst, Beijing 100084, Peoples R ChinaLi, Zheng论文数: 0 引用数: 0 h-index: 0机构: Tsinghua Univ, Dept Energy & Power Engn, State Key Lab Power Syst, Beijing 100084, Peoples R China Tsinghua Univ, Dept Energy & Power Engn, State Key Lab Power Syst, Beijing 100084, Peoples R ChinaWang, Zhe论文数: 0 引用数: 0 h-index: 0机构: Tsinghua Univ, Dept Energy & Power Engn, State Key Lab Power Syst, Beijing 100084, Peoples R China Tsinghua Univ, Dept Energy & Power Engn, State Key Lab Power Syst, Beijing 100084, Peoples R China
- [8] Spectral knowledge-based regression for laser-induced breakdown spectroscopy quantitative analysis[J]. EXPERT SYSTEMS WITH APPLICATIONS, 2022, 205Song, Weiran论文数: 0 引用数: 0 h-index: 0机构: Tsinghua Univ, Dept Energy & Power Engn, State Key Lab Power Syst, Int Joint Lab Low Carbon Clean Energy Innovat, Beijing 100084, Peoples R China Tsinghua Univ, Dept Energy & Power Engn, State Key Lab Power Syst, Int Joint Lab Low Carbon Clean Energy Innovat, Beijing 100084, Peoples R ChinaAfgan, Muhammad Sher论文数: 0 引用数: 0 h-index: 0机构: Tsinghua Univ, Dept Energy & Power Engn, State Key Lab Power Syst, Int Joint Lab Low Carbon Clean Energy Innovat, Beijing 100084, Peoples R China Tsinghua Univ, Dept Energy & Power Engn, State Key Lab Power Syst, Int Joint Lab Low Carbon Clean Energy Innovat, Beijing 100084, Peoples R ChinaYun, Yong-Huan论文数: 0 引用数: 0 h-index: 0机构: Hainan Univ, Coll Food Sci & Engn, Haikou 570228, Hainan, Peoples R China Tsinghua Univ, Dept Energy & Power Engn, State Key Lab Power Syst, Int Joint Lab Low Carbon Clean Energy Innovat, Beijing 100084, Peoples R ChinaWang, Hui论文数: 0 引用数: 0 h-index: 0机构: Queens Univ Belfast, Sch Elect Elect Engn & Comp Sci, Belfast BT9 5BN, Antrim, North Ireland Tsinghua Univ, Dept Energy & Power Engn, State Key Lab Power Syst, Int Joint Lab Low Carbon Clean Energy Innovat, Beijing 100084, Peoples R ChinaCui, Jiacheng论文数: 0 引用数: 0 h-index: 0机构: Tsinghua Univ, Dept Energy & Power Engn, State Key Lab Power Syst, Int Joint Lab Low Carbon Clean Energy Innovat, Beijing 100084, Peoples R China Tsinghua Univ, Dept Energy & Power Engn, State Key Lab Power Syst, Int Joint Lab Low Carbon Clean Energy Innovat, Beijing 100084, Peoples R ChinaGu, Weilun论文数: 0 引用数: 0 h-index: 0机构: Tsinghua Univ, Dept Energy & Power Engn, State Key Lab Power Syst, Int Joint Lab Low Carbon Clean Energy Innovat, Beijing 100084, Peoples R China Tsinghua Univ, Dept Energy & Power Engn, State Key Lab Power Syst, Int Joint Lab Low Carbon Clean Energy Innovat, Beijing 100084, Peoples R ChinaHou, Zongyu论文数: 0 引用数: 0 h-index: 0机构: Tsinghua Univ, Dept Energy & Power Engn, State Key Lab Power Syst, Int Joint Lab Low Carbon Clean Energy Innovat, Beijing 100084, Peoples R China Tsinghua Univ, Dept Energy & Power Engn, State Key Lab Power Syst, Int Joint Lab Low Carbon Clean Energy Innovat, Beijing 100084, Peoples R ChinaWang, Zhe论文数: 0 引用数: 0 h-index: 0机构: Tsinghua Univ, Dept Energy & Power Engn, State Key Lab Power Syst, Int Joint Lab Low Carbon Clean Energy Innovat, Beijing 100084, Peoples R China Tsinghua Univ, Dept Energy & Power Engn, State Key Lab Power Syst, Int Joint Lab Low Carbon Clean Energy Innovat, Beijing 100084, Peoples R China
- [9] Incorporating domain knowledge into machine learning for laser-induced breakdown spectroscopy quantification[J]. SPECTROCHIMICA ACTA PART B-ATOMIC SPECTROSCOPY, 2022, 195Song, Weiran论文数: 0 引用数: 0 h-index: 0机构: Tsinghua Univ, Dept Energy & Power Engn, Int Joint Lab Low Carbon Clean Energy Innovat, State Key Lab Power Syst, Beijing 100084, Peoples R China Tsinghua Univ, Dept Energy & Power Engn, Int Joint Lab Low Carbon Clean Energy Innovat, State Key Lab Power Syst, Beijing 100084, Peoples R ChinaHou, Zongyu论文数: 0 引用数: 0 h-index: 0机构: Tsinghua Univ, Dept Energy & Power Engn, Int Joint Lab Low Carbon Clean Energy Innovat, State Key Lab Power Syst, Beijing 100084, Peoples R China Tsinghua Univ, Shanxi Res Inst Clean Energy, Taiyuan 030032, Peoples R China Tsinghua Univ, Dept Energy & Power Engn, Int Joint Lab Low Carbon Clean Energy Innovat, State Key Lab Power Syst, Beijing 100084, Peoples R ChinaGu, Weilun论文数: 0 引用数: 0 h-index: 0机构: Tsinghua Univ, Dept Energy & Power Engn, Int Joint Lab Low Carbon Clean Energy Innovat, State Key Lab Power Syst, Beijing 100084, Peoples R China Tsinghua Univ, Dept Energy & Power Engn, Int Joint Lab Low Carbon Clean Energy Innovat, State Key Lab Power Syst, Beijing 100084, Peoples R ChinaAfgan, Muhammad Sher论文数: 0 引用数: 0 h-index: 0机构: Tsinghua Univ, Dept Energy & Power Engn, Int Joint Lab Low Carbon Clean Energy Innovat, State Key Lab Power Syst, Beijing 100084, Peoples R China Tsinghua Univ, Dept Energy & Power Engn, Int Joint Lab Low Carbon Clean Energy Innovat, State Key Lab Power Syst, Beijing 100084, Peoples R ChinaCui, Jiacheng论文数: 0 引用数: 0 h-index: 0机构: Tsinghua Univ, Dept Energy & Power Engn, Int Joint Lab Low Carbon Clean Energy Innovat, State Key Lab Power Syst, Beijing 100084, Peoples R China Tsinghua Univ, Dept Energy & Power Engn, Int Joint Lab Low Carbon Clean Energy Innovat, State Key Lab Power Syst, Beijing 100084, Peoples R ChinaWang, Hui论文数: 0 引用数: 0 h-index: 0机构: Queens Univ Belfast, Sch Elect, Elect Engn & Comp Sci, Belfast BT9 5BN, North Ireland Tsinghua Univ, Dept Energy & Power Engn, Int Joint Lab Low Carbon Clean Energy Innovat, State Key Lab Power Syst, Beijing 100084, Peoples R ChinaWang, Yun论文数: 0 引用数: 0 h-index: 0机构: Univ Calif Irvine, Dept Mech & Aerosp Engn, Renewable Energy Resources Lab RERL, Irvine, CA 92697 USA Tsinghua Univ, Dept Energy & Power Engn, Int Joint Lab Low Carbon Clean Energy Innovat, State Key Lab Power Syst, Beijing 100084, Peoples R ChinaWang, Zhe论文数: 0 引用数: 0 h-index: 0机构: Tsinghua Univ, Dept Energy & Power Engn, Int Joint Lab Low Carbon Clean Energy Innovat, State Key Lab Power Syst, Beijing 100084, Peoples R China Tsinghua Univ, Shanxi Res Inst Clean Energy, Taiyuan 030032, Peoples R China Tsinghua Univ, Dept Energy & Power Engn, Int Joint Lab Low Carbon Clean Energy Innovat, State Key Lab Power Syst, Beijing 100084, Peoples R China
- [10] Industrial at-line analysis of coal properties using laser-induced breakdown spectroscopy combined with machine learning[J]. FUEL, 2021, 306 (306)Song, Weiran论文数: 0 引用数: 0 h-index: 0机构: Tsinghua Univ, Dept Energy & Power Engn, State Key Lab Power Syst, Int Joint Lab Low Carbon Clean Energy Innovat, Beijing 100084, Peoples R China Tsinghua Univ, Dept Energy & Power Engn, State Key Lab Power Syst, Int Joint Lab Low Carbon Clean Energy Innovat, Beijing 100084, Peoples R ChinaHou, Zongyu论文数: 0 引用数: 0 h-index: 0机构: Tsinghua Univ, Dept Energy & Power Engn, State Key Lab Power Syst, Int Joint Lab Low Carbon Clean Energy Innovat, Beijing 100084, Peoples R China Tsinghua Univ, Shanxi Res Inst Clean Energy, Taiyuan 030032, Shanxi, Peoples R China Tsinghua Univ, Dept Energy & Power Engn, State Key Lab Power Syst, Int Joint Lab Low Carbon Clean Energy Innovat, Beijing 100084, Peoples R ChinaGu, Weilun论文数: 0 引用数: 0 h-index: 0机构: Tsinghua Univ, Dept Energy & Power Engn, State Key Lab Power Syst, Int Joint Lab Low Carbon Clean Energy Innovat, Beijing 100084, Peoples R China Tsinghua Univ, Dept Energy & Power Engn, State Key Lab Power Syst, Int Joint Lab Low Carbon Clean Energy Innovat, Beijing 100084, Peoples R ChinaWang, Hui论文数: 0 引用数: 0 h-index: 0机构: Ulster Univ, Sch Comp, Newtownabbey BT37 0QB, North Ireland Tsinghua Univ, Dept Energy & Power Engn, State Key Lab Power Syst, Int Joint Lab Low Carbon Clean Energy Innovat, Beijing 100084, Peoples R ChinaCui, Jiacheng论文数: 0 引用数: 0 h-index: 0机构: Tsinghua Univ, Dept Energy & Power Engn, State Key Lab Power Syst, Int Joint Lab Low Carbon Clean Energy Innovat, Beijing 100084, Peoples R China Tsinghua Univ, Dept Energy & Power Engn, State Key Lab Power Syst, Int Joint Lab Low Carbon Clean Energy Innovat, Beijing 100084, Peoples R ChinaZhou, Zhenhua论文数: 0 引用数: 0 h-index: 0机构: Guodian Zhuanghe Power Dev Co Ltd, Dalian 116400, Liaoning, Peoples R China Tsinghua Univ, Dept Energy & Power Engn, State Key Lab Power Syst, Int Joint Lab Low Carbon Clean Energy Innovat, Beijing 100084, Peoples R ChinaYan, Gangyao论文数: 0 引用数: 0 h-index: 0机构: Guoneng Bengbu Power Generat Co Ltd, Bengbu 233412, Anhui, Peoples R China Tsinghua Univ, Dept Energy & Power Engn, State Key Lab Power Syst, Int Joint Lab Low Carbon Clean Energy Innovat, Beijing 100084, Peoples R ChinaYe, Qing论文数: 0 引用数: 0 h-index: 0机构: Guoneng Bengbu Power Generat Co Ltd, Bengbu 233412, Anhui, Peoples R China Tsinghua Univ, Dept Energy & Power Engn, State Key Lab Power Syst, Int Joint Lab Low Carbon Clean Energy Innovat, Beijing 100084, Peoples R ChinaLi, Zhigang论文数: 0 引用数: 0 h-index: 0机构: Jinneng Holding Shanxi Elect Power Tashan Power G, Datong 037000, Shanxi, Peoples R China Tsinghua Univ, Dept Energy & Power Engn, State Key Lab Power Syst, Int Joint Lab Low Carbon Clean Energy Innovat, Beijing 100084, Peoples R ChinaWang, Zhe论文数: 0 引用数: 0 h-index: 0机构: Tsinghua Univ, Dept Energy & Power Engn, State Key Lab Power Syst, Int Joint Lab Low Carbon Clean Energy Innovat, Beijing 100084, Peoples R China Tsinghua Univ, Dept Energy & Power Engn, State Key Lab Power Syst, Int Joint Lab Low Carbon Clean Energy Innovat, Beijing 100084, Peoples R China